Our minisymposium proposal has been accepted. We will provide the information in this web page.
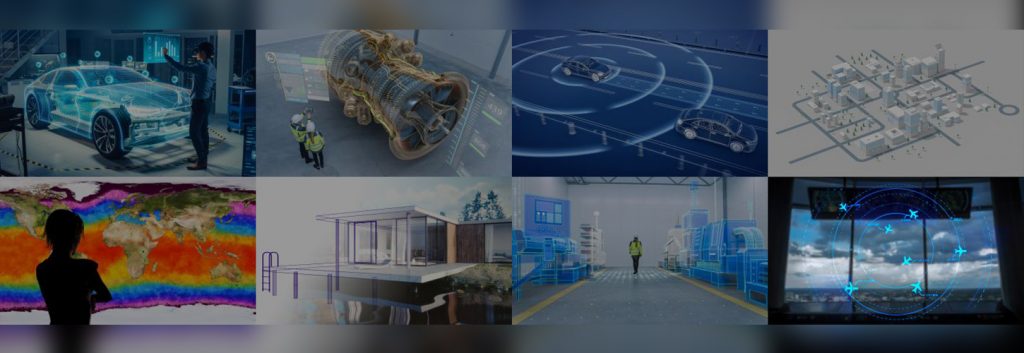
Summary
Traditionally, simulation and machine learning have had opposite research directions: deductive and inductive computations. Many types of simulations based on the knowledge in their application fields have been developed and provided interpretable analysis in data mining, while in the filed of machine learning the development of surrogate model technology by deep learning and Gaussian process allows us to approximate the system in the real world as a black box with high accuracy.
In recent years, new research has been expanding based on methods and models of the opponent in each field; simulation has high accuracy and its cost is reduced by using a surrogate model and data assimilation techniques, and new research problems of machine learning are discovered by embedding simulators and first-principles calculations in a part of the statistical models.
However, because research issues tend to depend on the simulation application domain, the results are limited in each field. In this mini-symposium, we introduce simulation methods based on machine learning and machine-learning methods based on simulations, and explore possibility of an essential fusion of the fields. Particularly the intersection of simulation optimization and statistical modeling is considered through data assimilation. We also build a community by providing a place to effectively share the issues and the achievements across the fields.
Target audience
This minisymposium aims to attract all researchers and professionals of data mining and machine learning, who are interested in simulations, and researchers and engineers of simulations, who try to use techniques of machine learning and statistics.
Program
(Affiliation and position are as of the time of the event.)
- 1. opening & welcome
- by Keisuke Yamazaki
10 mins
▼
TBA
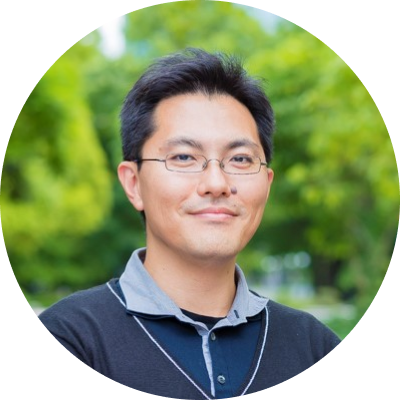
- 2. invited talk 1
by Dr. Keiichi Kisamori
▼
Dr. Keiichi Kisamori
Principal of BIRD INITIATIVE Inc.
k-kisamori@bird-initiative.com
https://bird-initiative.com/about-us/
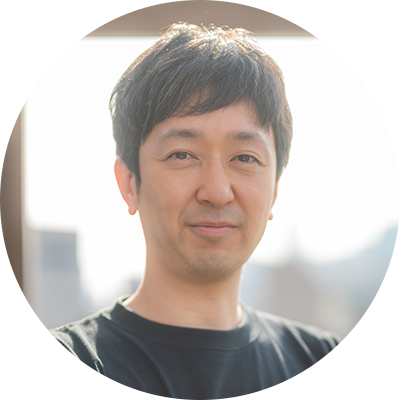
- 3. Knowledge-Guided Machine Learning: A New Framework for Accelerating Scientific Discovery
- by Professor Vipin Kumar
▼
Professor Vipin Kumar
University of Minnesota
This talk makes a case that in a real-world systems that are governed by physical processes, there is an opportunity to take advantage of fundamental scientific knowledge to inform the search of a physically meaningful and accurate ML model. While this talk will illustrate the potential of the knowledge-guided machine learning (KGML) paradigm in the context of environmental problems (e.g., Fresh water science, Hydrology, Agroecology), the paradigm has the potential to greatly advance the pace of discovery in a diverse set of discipline where mechanistic models are used, e.g., power engineering, climate science, weather forecasting, and pandemic management
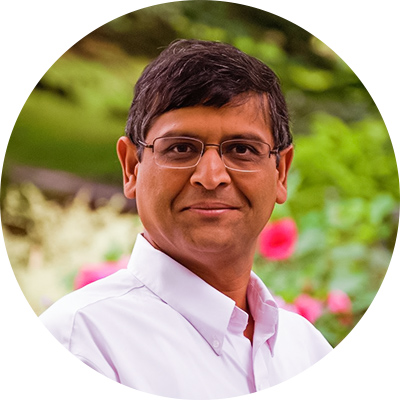
- 4. Optimizing simulation models through surrogate Machine learning models
- TBA
▼
Ruben Peters
Senior Data Scientist at Royal HaskoningDHV
One of the hardest aspects of simulation models is how to optimize the input parameters. Generally, this can be a very costly and time-consuming process. To overcome this problem, we have trained a Gaussian Process model on the output and input of Lanner’s WITNESS simulation program and used Bayesian Optimization to quickly suggest better simulation scenarios that can then be checked by WITNESS. This iterative approach allows the simulation modelers to quickly find better scenarios while still using the simulation model to check for feasible solutions.
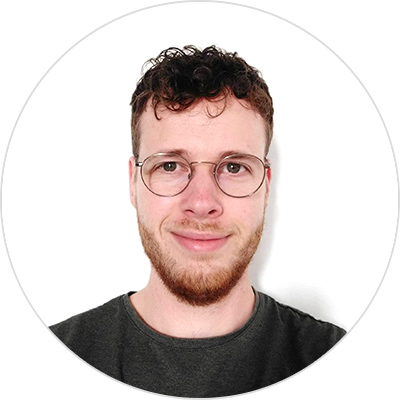
- 5. Towards Integration of Data Assimilation and Deep Learning Beneficial to Seismology
- by Professor Hiromichi Nagao
▼
Professor Hiromichi Nagao
Earthquake Research Institute, The University of Tokyo
Data assimilation, which integrates numerical simulations and observational data based on Bayesian statistics, has been widely applied in seismology. Deep learning is expected to make data assimilation much more effective even when seismological numerical simulations are massive. We introduce our works such as development of a new data assimilation algorithm, implementation of the replica exchange Monte Carlo on data assimilation to simultaneously estimate seismic wave propagations and underground structures, application of a convolutional neural network to extract seismic phenomena from images, and current trials towards integration of data assimilation and deep learning aiming in the national seismological projects in Japan.
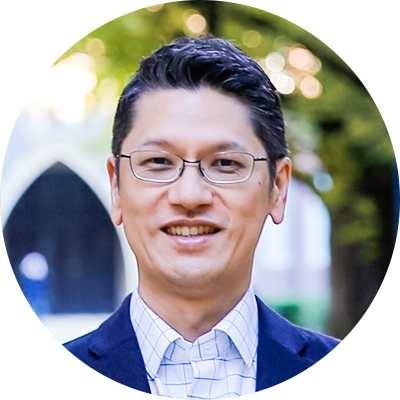
- 6. Statistical Machine Learning for Materials Modeling and Simulation
- by Professor Ryo Yoshida
▼
Professor Ryo Yoshida
The Institute of Statistical Mathematics, Research Organization of Information and Systems
This talk will describe the potential of integrating machine learning and simulation in materials science. The most significant barrier to implementing data-driven materials research stems from the lack of sufficient amounts of data. In addition, the ultimate goal of materials research is to discover innovative materials that exist in unexplored areas where no data exist. Therefore, interpolative predictions using fully data-driven approaches are generally not sufficient to achieve this goal, and the integration of computer experiments into a machine learning pipeline plays an important role in materials science. This talk will present a case study of materials exploration based on transfer learning and adaptive design of experiments on a high-dimensional design space.
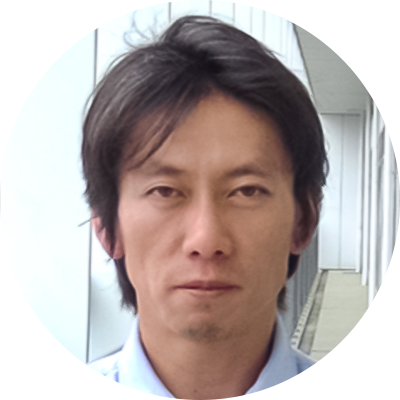
- 7. closing remarks
- by Keisuke Yamazaki
5 mins
▼
TBA
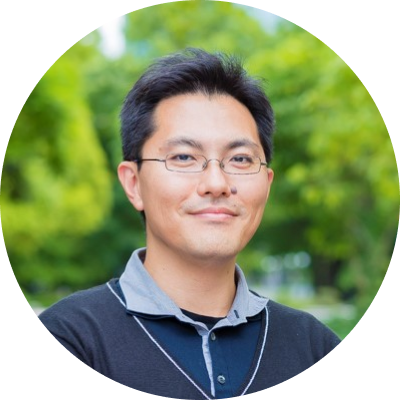
Organizer
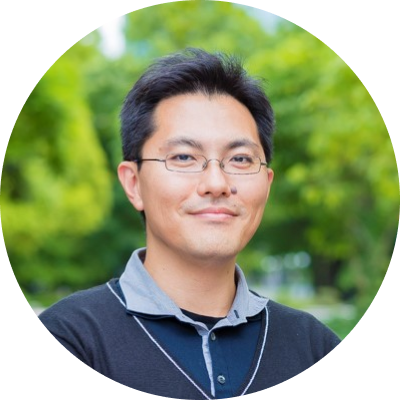
Keisuke Yamazaki
BIRD INITIATIVE Inc.
k.yamazaki@bird-initiative.com
■Biography of organizer
Keisuke Yamazaki is the leader of machine learning research team in National Institute of Advanced Industrial Science and Technology, and also working in BIRD-INITIATIVE Inc. His research interest focuses specifically on the Bayesian statistics with algebraic geometry and its connection to simulation algorithm. His papers have been published in journals and international conferences of machine learning such as Neural Networks, JMLR, Machine Learning, ICML and AISTATS. He is now proposing an organized session in the annual conference of Japanese Society of AI to provide a place to share the issues in machine learning and simulation.